Artificial intelligence, drones, and robots are already being deployed on large farms to assist with several farm management tasks for crops and livestock. However, there are some risks that must be accounted for when turning over our food production to AI-driven machines.
We will discuss the benefits that AI can bring to the world of agriculture, including some applications that are already in place to help our farmers produce more and better-quality food. We will then discuss some potential pitfalls we must be aware of if we turn over our food supply to machines.
AI’s Potential
AI has brought to the world countless tools for personal and industrial use. With agriculture, it has delivered the potential to increase yields, keep pests away, and reduce costs in nearly all parts of farm management.
Our farmers need to know how best to use these tools, and we need to understand how their application can be a benefit. There are already AI applications that are worthwhile and are providing users with successful results. Let us see how the grass is greener on the AI side.
The Smart Farm
AI is leading to smart farms with farming models that have high cognitive ability. This technology is focused on a few specific areas.
Data and Analysis
With new equipment, farms can be set up to track and analyze multiple data points. For example, a farmer can use a drone to review a large tract of land and identify the exact location of a pest infestation or plant disease in real-time. This mass of data has boosted information accuracy and can help farmers make informed decisions when analyzed with AI models.
Robotics and Automation
Robots are used for farm activities such as picking, thinning, and sorting to speed up manual labor work and deal with any labor shortages. The goal is to increase productivity, consistency, and quality while minimizing errors.
Predictions
AI models have been designed to predict changes to weather patterns, soil erosion, and pest infestations to improve farm management and planning. These tools allow farmers to see into the future, assisting them with informed decision-making.
Like other industries, agriculture faces similar constraints related to its use of AI, such as compatibility with current technology, resource availability, security, and potential regulatory issues. Even with these constraints, the future farms will be highly dependent on AI, making them more precise and creating a new “cognitive farm.”
Digital Farmers
AI is revolutionizing one of our oldest industries and giving farmers multiple ways to produce more abundant harvests in all parts of the world. With this transformation, farms will now require digital farmers, men and women, which can push forward these technological changes, managing future farms in new ways.
Tools and People
New farm managers must understand and use the correct tools to their farm’s benefit. While extensive technical knowledge is not needed, understanding the basic principles behind the technology and, more importantly, the technology’s operational implications are necessary. Through AI, farm managers can better understand the inner workings of their farms.
The changing technology means that farm talent must be updated. Beyond the typical farming roles, farms will require employees with technological skills. The entire organization will need defined education to stay on top of the AI farming future.
New Ways of Farming
Farmers will need to leave their comfort zones and explore new collaborative opportunities. This change will involve collaboration with new companies to obtain cutting-edge technologies that will allow a farm to acquire a competitive advantage and boost productivity. These partnerships provide inimitable technologies, giving farmers the upper hand, but these technologies work best for large farms.
Cost advantages are most significant with economies of scale. So, managers will benefit by finding strength in numbers. AI tools can be expensive, beyond the reach of the small farm. When collaborating with other farms, cooperatives, suppliers, universities, local communities, and the government, these costs can be driven down.
AI’s Current Applications
AI currently monitors soil, detects pests, determines diseases, and applies intelligent spraying. Here are a few of the current applications farmers are already using today.
Crop Monitoring
Crop health relies on micro and macronutrients in the soil to produce yields but with quantity and quality. Once the crops are planted, monitoring their growth to optimize production is also needed. Understanding the interaction between growth and the environment is vital to adjust for healthy crops. Traditionally this was done through human observation and experience, but this method is neither accurate nor speedy.
Now drones capture aerial data, then train computer models to intelligently monitor crops and soil. This AI system can use the collected data to:
- Track the health of the crops
- Accurately predict yields
- Identify crop malnutrition
This can all be done faster than a human could, in real-time, providing farmers with specific problem areas so they can take immediate actions to prevent problems before they grow.
Determining Crop Maturity
Wheat head growth is a labor-intensive process that can be aided with AI. Over a three-year period, researchers collected wheat head images at different stages with different lighting, building a two-step wheat ear detection system. The AI model was able to outperform human observation, allowing farmers not to have to make daily visits to fields to check on the crops.
Similarly, tomato ripeness has been determined with AI.
A different study examined how well AI can detect maturity in tomatoes. The researchers built a model looking at the color of five different parts of a tomato, then made maturity estimates. The algorithm could correctly classify tomatoes with a 99.31% accuracy.
Generally, evaluating soil involves digging up samples and sending them to the lab for analysis. AI researchers have used image data from a cheap microscope to train their model to do the same task. The model was able to make sand content and soil organic matter estimates with accuracy similar to costly and slower lab analyses.
Disease and Insect Detection
Using deep learning, farmers are now automating the detection of plant diseases and pests. This is done through image classification and segmentation.
A study looked at the apple black rot and used a deep neural network AI model to identify the four stages of disease severity. Like with the other models above, the disease identification process is labor-intensive. This project was able to identify the disease severity at an accuracy of 90.4%.
Similarly, a different study was able to use the YOLO v3 algorithm and was able to identify multiple pests and diseases on tomato plants. Using only a digital camera and smartphone, researchers identified twelve different cases of disease or pests. Once trained, it was able to detect problems with an accuracy of 92.39%, taking only 20.39 milliseconds.
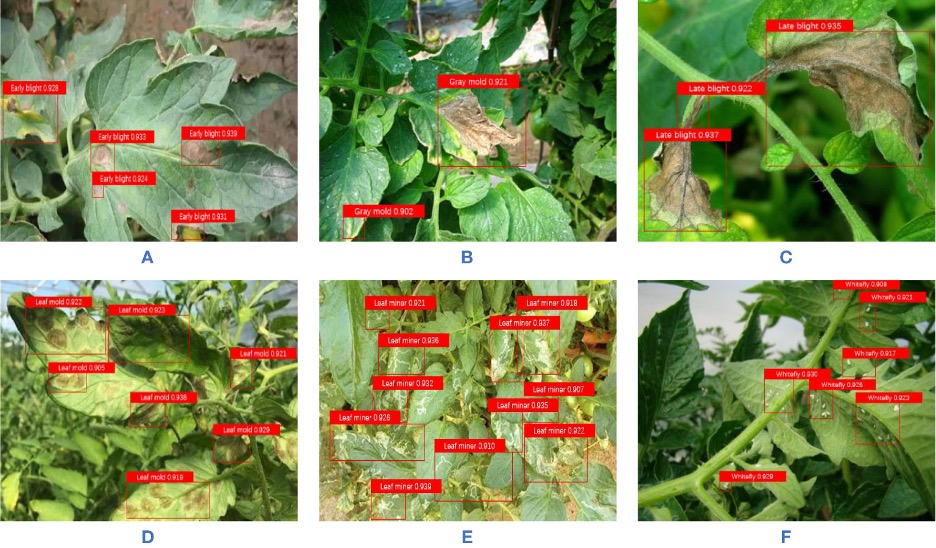
Another study used sticky traps to collect six flying insects and collect images. They then based the course counting on object detection and fine-counting results. The model identified bees, mosquitoes, moths, flies, chafers, and fruit flies with a 90.18% accuracy and a 92.5% counting accuracy.
Livestock Monitoring
Animals are a major component of our food system and need even more tracking than plants. Companies are now offering tools to track cattle and chickens. CattleEye tracks and annotates key points for individual cows.
The system uses overhead cameras to monitor animal health and behavior, allowing a rancher to spot a problem and be notified without being next to the cow.
By collecting data with cameras and drones, this kind of software is being used to count animals, detect disease, monitor birthing, and identify unusual behavior. It also confirms access to food and water.
Smart Spraying
AI also prevents problems in the first place. Drones help with the spraying of fertilizer and pesticides uniformly across a field. They operate with high precision in real-time, spraying correctly and reducing contamination risk to animals, humans, and water resources.
This is a growing field and is best performed by multiple drones, but intelligent spraying is getting better. Virginia Tech researchers developed a smart spray system that can detect weeds.
A camera mounted on a sprayer records the geolocation of the weeds, analyzing their size, shape, and color, and then delivers a precise amount of herbicide.
The device’s accuracy prevents collateral damage to other crops in the environment.
Risks of AI in Agriculture
All these different AI applications will help us monitor and improve our food systems, helping feed the 2.4 billion people suffering from food insecurity. AI can reduce labor inefficiency and increase reliability. However, there are some cautionary tales.
According to a release by Asaf Tzachor of Cambridge University, there could be flaws in the agricultural data, emphasizing productivity over environmental concern. This focus could lead to errors that cause over-fertilization and pesticide use, improper irrigation, and soil erosion. These factors must also be considered when designing AI systems. Inadvertent changes resulting in crop failures could result in massive food insecurity.
Cybersecurity is a second issue. Cyberattacks could disrupt entire food systems, especially for farms that rely heavily on AI.
Finally, those without access to the new technology could be cut out of markets. Big farmers will profit, and small farms will be locked out of the gains entirely if they cannot afford the AI infrastructure.
Planning Ahead
As in all enterprises, diligence and conscientious planning contribute to farming success. Farmers must plan their AI strategy by optimizing their operations and yield requires thoughtful assessment. This planning involves a thorough review of priorities and a clear implementation plan.
AI provides tools that can boost a farm’s yields, and transform the industry. Increases in agricultural production on a large scale will impact a country’s GDP, increase food security, and positively impact the environment. The US had just over two million farms in 2021, averaging 445 acres each, totaling 89.5 million across the country.
Analytics and robotics boosts production on almost any farm. AI-related productivity gains can reshape the farming business and improve our global food supply. This is a way we can counteract the climate factors that could affect corn, rice, soy, and wheat production by 20-49%.
Closing Thoughts
Since the advent of agriculture, technology has improved its efficiency. From plows and irrigation to tractors and AI, we have moved forward to feed our growing population. With the ongoing changes to our climate, AI has arrived just in time to save us all from potential food insecurity. We must use AI to increase efficiency and reduce food production costs while also improving environmental sustainability. Doing so can make our farmers “smarter” and give us more and healthier foods.
If small farmers can work together and take full advantage of these new AI tools, they can compete with large industrial farms. We also have to ensure that the systems that are put into place are safe and have an all-encompassing view that does not only focus on yields but the potential environmental effects. Sustainability remains crucial, and AI is the missing piece.
Disclaimer: The information provided in this article is solely the author’s opinion and not investment advice – it is provided for educational purposes only. By using this, you agree that the information does not constitute any investment or financial instructions. Do conduct your own research and reach out to financial advisors before making any investment decisions.
The author of this text, Jean Chalopin, is a global business leader with a background encompassing banking, biotech, and entertainment. Mr. Chalopin is Chairman of Deltec International Group, www.deltecbank.com.
The co-author of this text, Robin Trehan, has a bachelor’s degree in economics, a master’s in international business and finance, and an MBA in electronic business. Mr. Trehan is a Senior VP at Deltec International Group, www.deltecbank.com.
The views, thoughts, and opinions expressed in this text are solely the views of the authors, and do not necessarily reflect those of Deltec International Group, its subsidiaries, and/or its employees.
One thought on “AI in Agriculture ”
Comments are closed.