Imagine a time before Facebook (Meta) and Google, way back in the middle of the dot.com bubble. The real inventor of the World Wide Web, Tim Berners-Lee, coined the term Semantic Web in 1999. It represents a web of data being processed by machines, especially since the data is uniquely machine-readable. Stating in his book, Weaving the Web:
I have a dream for the Web [in which computers] become capable of analyzing all the data on the Web – the content, links, and transactions between people and computers. A “Semantic Web,” which makes this possible, has yet to emerge, but when it does, the day-to-day mechanisms of trade, bureaucracy, and our daily lives will be handled by machines talking to machines. The “intelligent agents” people have touted for ages will finally materialize.”
His vision was better outlined in a 2001 Scientific American article, where Berners-Lee described an evolution of the (then) current Web to a Semantic Web. The Semantic Web, or “Web 3.0,” is an extension of the World Wide Web with its standards by the W3C. Because the Metaverse has taken the moniker of Web 3.0, the Semantic Web has been relegated to Web 4.0.
Critics continue to question Semantic Web’s feasibility. Proponents argue that several applications remain proven, and the original concept is valid. We shall discuss the current and future potential of the Semantic Web.
What Is the Semantic Web?
So beyond “machine-readable,” what does the term mean? The definition of the Semantic Web differs significantly from person to person, but for our purposes, the Semantic Web is a virtual environment in which information and data are arranged so that they are processed automatically.
The machines then read content. Meaning, they interpret data automatically. Artificial intelligence (AI) functionality derives itself from these automated machines, now enabling users to interact with them in a “human” way.
The machines’ goal is to replicate the experience of engaging with another person. They interpret your data, your meaning, through bodily actions, words, or clicks. Two great examples here are Alexa and Siri, both programmed to record your preferences.
The Semantic Web’s Ongoing Evolution
The foundation of this evolution depends upon data: sharing, discovery, integration, and reuse.
One of its main components is the creation of ontologies. The Web is a web: the components draw connections from each other and exist as a compilation of interlinked units. Starting in 2006, “RDF” (Resources Description Framework) graphs were used to make data sharing organized.
Ontology: a set of concepts and categories in a subject area which shows their properties and their interrelations.
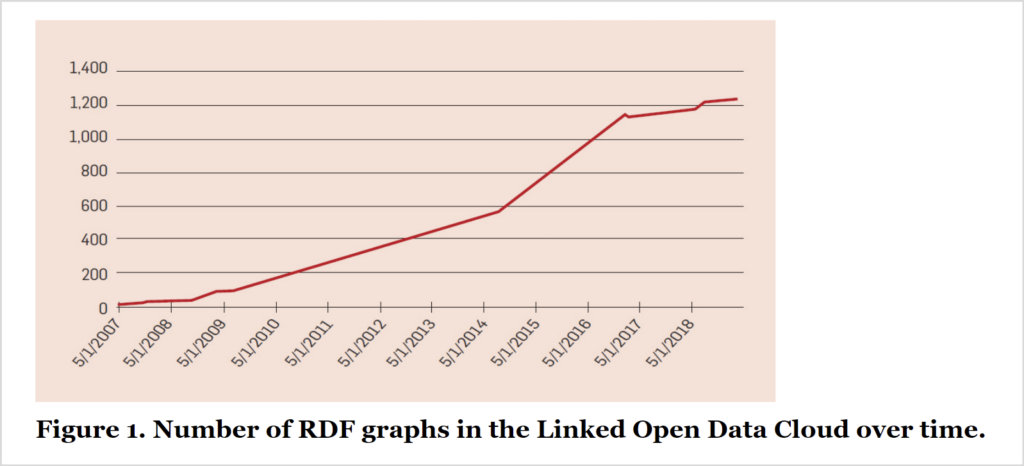
Graph courtesy of communications of the ACM
The Linked Open Data Cloud’s RDF graphs represent only a small portion of the 650,000 data documents being used in the ongoing research of ontologies.
After combining ontologies, it was discovered that they possessed significant limitations. General interest in linked data waned as researchers discovered that utilizing data required much more of it. The result: knowledge graphs, presenting ready-to-share data efficiently.

Image courtesy of Towards Data Science
From this moment, the inner workings of the Semantic Web grew to be increasingly complicated. It is not driven by certain methods inherent to the field, distinguishing it from other data-related areas such as machine learning–which is more focused and easier to improve.
The Semantic Web is foremost a conceptual vision: that all components far and wide should speak to one another in the same language. Its broad mission but lack of specific focus means it is far less organized than more widely accepted innovations.
What is Web 4.0?
A good analog is an umbrella. It must combine augmented reality with distributed tech with Big Data, or the major components of Web 3.0, in an overarching web, pun intended. This linking is the essence of Web 4.0.
Users will have their own avatars, or digital alter egos, for interacting with AI or humans. AI digital assistants do not only respond to requests but remain proactive.
Let’s take a simple example. You’re on the way to LaGuardia airport, and your driverless Uber is stuck in traffic. Your digital assistant will inform you that with the current traffic patterns, and you’re going to miss your booked flight. However, the assistant already pre-booked a different flight out of JFK airport and can automatically send you there. It changes the route of your Uber, while also informing your family that you will be home only 15 minutes later than expected. All this would be done after receiving your initial “okay.”
Some may see this is great, while others find it a dystopian future where there is too much access and control over your information.
Challenges
Despite the advances going into 2022, the Semantic Web remains difficult to implement given current technology. Computers do not yet fully understand the nuances of human languages, such as tones, mannerisms, phrases, changing in pitch, and so on.
Specific challenges the Semantic Web must contend with are deceit, inconsistency, uncertainty, vagueness, and vastness. Any system will need to effectively deal with all these issues simultaneously.
Deceit: when the information’s producer intentionally misleads its consumer. Cryptography does reduce this threat. However, additional processes supporting information integrity, or lack thereof, are required.
Inconsistency: when information from separate sources is combined, resulting in contradictions in logic, flow, or meaning. The deductive reasoning employed by computers fall apart when “anything follows from a contradiction.” Two techniques known to deal with this inconsistency are defeasible reasoning and paraconsistent reasoning.
Uncertainty: computers don’t like precise concepts with uncertain values. Rather, one should be one, nor two or three too. For example, a medical patient might present symptoms belonging to a range of possible diagnoses. Uncertainties such as these can be addressed with probabilistic reasoning.
Vagueness: imprecise questions such as, “how many grains of sand make up a pile?” or even concepts like tall and young are complex for a computer to deal with efficiently; everyone has their definition. Matching query terms with different knowledge bases that provide overlapping but subtly different concepts help. Fuzzy logic is as an additional remedy for the issue of vagueness.
Vastness: With billions of pages on the Web already, it’s difficult to determine what is specifically needed for certain contexts. SNOMED CT dictionary has 370,000 terms, a relatively small amount, yet the existing system has been unable to eliminate semantically duplicate terms. Future automated reasoning systems will have to deal with inputs on the level of billions or trillions.
While this list does not cover all the issues in creating the Semantic Web, it demonstrates the most critical challenges to be solved first.
The World Wide Web Consortium’s Incubator Group for Uncertainty (URW3-XG) lumps these problems all together in their report under a single heading, “uncertainty.” The techniques of possible solutions will require an extension to the Web Ontology Language (OWL) to, for example, annotate conditional probabilities. This is an area of ongoing research which is yet to “solve” anything yet.
Feasibility of the Semantic Web
Companies which have historically invested in the Semantic Web for decades are still hurdles in bringing it to life. Recently, IBM sold off much of its Watson Health program. Sadly, many of the same problems affecting the Semantic Web 20 years ago remain.
- Scalability
- Multilinguality
- Reducing information overload with visualization
- Semantic Web language stability
The Semantic Web’s promise virtually ensures mainstream adoption, but not without more efficient data management solutions. AI remains away from reaching the point of human comprehension and interaction.
Summary
The potential of the Semantic Web is incredible. Semantics is a slow-moving field, and as new discoveries are made, even more pain points will be discovered. Yet we are making progress. Companies have spent fortunes on Semantic Web development and will continue to do so. It will eventually happen. There is a light at the end of the tunnel. We just don’t know the length of that tunnel.
Disclaimer: The author of this text, Jean Chalopin, is a global business leader with a background encompassing banking, biotech, and entertainment. Mr. Chalopin is Chairman of Deltec International Group, www.deltecbank.com.
The co-author of this text, Robin Trehan, has a bachelor’s degree in economics, a master’s in international business and finance, and an MBA in electronic business. Mr. Trehan is a Senior VP at Deltec International Group, www.deltecbank.com.
The views, thoughts, and opinions expressed in this text are solely the views of the authors, and do not necessarily reflect those of Deltec International Group, its subsidiaries, and/or its employees.